Why it's time for 'Attention Version 2' — the science of attention
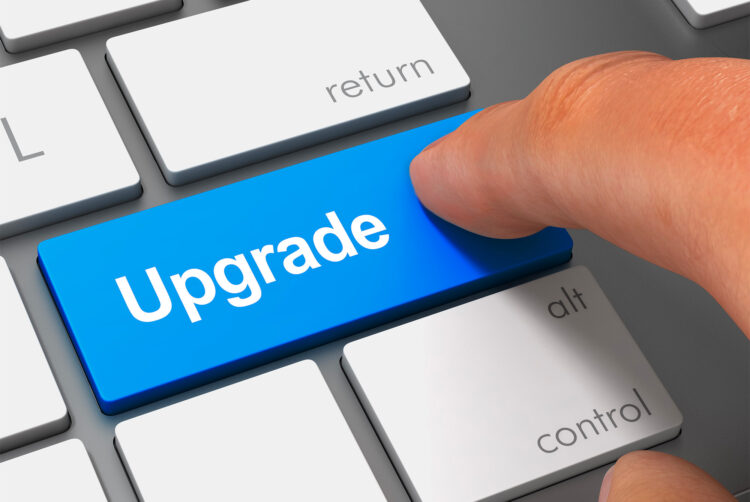
Opinion: Attention Revolution
‘Good enough’ is not an option over excellence. It’s time to graduate to the next level.
It has been 12 months since I started writing Attention Revolution for The Media Leader, over which time we have penned a blueprint for an emerging industry.
The series started with big picture thinking from the scale of the currency problem to a description of how the product ecosystem will likely land. We evolved to slightly deeper concepts over the year including guiding rules for quality attention data to the double jeopardy patterns obvious within it. And, along the way, we celebrated some wins as attention economics looked to be hitting critical mass adoption. A point very much quantified this year at Cannes Lions when I was told at one point during the week that “attention measurement is like crack to the advertising community”.
The level of change is an incredible achievement for our industry. And everyone should be proud, change is hard. But I look at the last 12 months as Version 1 of the attention journey. A year where we learned mostly basics, with only a couple of hard tests.
Starting with basics was fine because, had we overcomplicated Year 1 it may have set us back — just as teaching final-year material to a first-year college student would increase stress and the likelihood of dropout.
While simple concepts have led us through these last 12 months, it’s time to graduate and you are ready. Today I call for the industry to transition from Attention V1 to Attention V2. A move from attention concepts to attention science.
Let me explain.
Attention concepts produce case evidence i.e. “We did X with Brand Y and it produced Z attention outcome.”
Case evidence is a result of a single moment in time. Change the conditions of a case study and the outcome will highly likely differ, yet replication of results is vital for applicable value in attention planning and buying.
If case studies can’t be replicated, and differences can’t be explained, their findings can’t be applied with confidence. A dice roll at best (mind you, case evidence has been handy to demonstrate surface level success stories for attention conversations).
Attention science, however, produces norms e.g. Attention Elasticity is the range of attention possible under the conditions of the platform and format. Attention Shapes are systematic viewing clusters based on a set of attention features that provide an algorithmic description of how information is integrated over time by different audiences.
Replication is at the core of these norms. Change the test conditions of these studies and these results will likely hold, and if there are differences they are explainable. Replication is the key to accuracy and is vital for applicable value in attention planning and buying.
Why does Attention Science matter?
Because if we don’t apply science we run the risk of simplistic applications doing more harm than good. ‘Good enough’ is where we’ve come from, and we have spent the last 10 years paying the price.
For example, we have learned that there is a lot of compounded error beneath ‘good enough’ attention models. We know that generalisable norms set the blueprint for successful attention prediction and without accounting for these patterns an attention model will be wrongly skewed by its own training parameters.
An example of this is the typical use of pixels, scroll speed, time on screen and ad coverage as predictive tags. Turns out these factors can be contributors to both attention and distraction. And making life harder, in cases where these factors are predictors of human attention, the individual contribution (weighting) of each factor varies by every single platform and format. Think of it like a cookie recipe where the baseline ingredients sometimes will be the same, sometimes they won’t, but even then the number of pounds or ounces needed by each ingredient might vary.
This is the complexity of human attention. And this is why a full screen video ad unit on one platform may deliver different amounts of attention than another platform. Or a 9×16 ad unit in one feed format may deliver different amounts of attention than another feed format. Both ad units are the same size, but sometimes these ad units render different results. And the cause of these differences may be nothing at all to do with ad size at all.
So when attention models are trained on device-based tags that have underlying variation in their capacity to predict human attention, it will compound error and the model’s predictive quality will get worse not better because the model learns unintentional data artefacts. When an attention model is continuously trained against human attention parameters, its accuracy will strengthen and consistent outcomes for the brand will prevail.
Why does any of this matter?
In the emerging category of attention measurement, not only do we have the power to avoid catastrophe (again), we also have the rare opportunity to create truly positive and long-lasting change. But the science of attention needs scientific rules. Rules around data quality, model accuracy and ethical practices. Generalisable norms go a long way to this effect, and what we have discussed today only scratches the surface.
To agencies and brands who are graduates of Attention V1, I leave you with this thought; good enough is not an option over excellence. Welcome to the next level in attention measurement.
Professor Karen Nelson-Field is a media science researcher and founder of Amplified Intelligence. Attention Revolution is a monthly column for The Media Leader in which she explores how brands can activate attention to measure online advertising as well as build a better digital ecosystem.