We need to talk about frequency
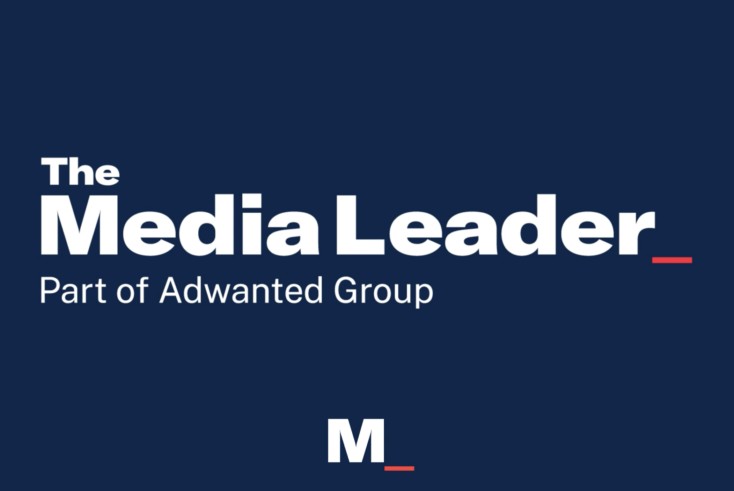
Sponsor content Measuring true frequency is very difficult, so what are the different approaches advertisers can take? AudienceProject’s Martyn Bentley investigates
A lot has been written about the complexity (and accuracy) of cross-device audience measurement in digital marketing, and a lot of claims are made by the players in the space. However, it is often discussed in the technical context of programmatic without too much of a reference to the actual meaning of this to marketers. And the meaning to those spending the money is really frequency.
Frequency is a crucial media planning tactic – it’s a word often used in TV planning and buying because frequency is an important optimisation lever in maximising media ROI, especially when large TV budgets are concerned.
A frequency of 1 arguably provides the greatest ROI possible – it is a binary “no, I’ve never heard of that product” against a “yes, I have now heard of that product”. If someone doesn’t know about your product, they can never buy it. You need to reach them, let them know it exists, and then start persuading them that they want or need it.
Higher frequencies can solidify brand familiarity, drive desire and intent, and move people through the funnel. But too high a frequency will start to annoy users and move your media budget beyond the point of diminishing returns (online retargeting, anyone!?). Obviously, marketers don’t want to waste money or annoy potential or existing customers.
So, with all the promise that online media tracking offers, it’s still incredibly difficult to measure real people across their various devices, and ergo establish the true frequency – and thus start to understand the true efficacy of your media budget on driving demand.
There are various approaches to (partially) solving the online cross-device or frequency problem – and as we know, some companies like Facebook who offers logged in users, have certain advantages. However, all is not lost if you are not Facebook, and of course, marketers spend across various online media, not just the ‘walled gardens’, so we all still need to go beyond that.
In this article, we look at why the problem of measuring true frequency is a hard one, different approaches to deal with the issue as well as the approach used by AudienceProject.
New world, new challenges
Today, we live in a world where people use an ever-increasing number of devices making it more difficult than ever to get the frequency right when measuring audiences.
Earlier, only a few big TV-stations existed and very few devices were available for watching TV, whereas the digital world today is characterized by multi-device usage and an extremely long tail of media in which campaigns are executed.
With this new media reality, proper audience measurement can only be achieved if you couple new technologies with panels that are much bigger than classical TV panels – which at the same time needs to be recruited diversely, weighted, managed properly and fused with information that is not only survey-based.
Why the problem is a hard one
The problem of measuring frequency correctly is often due to a lack of methodical understanding of the challenge that lies ahead and a lack of technical ability to de-duplicate from devices to humans.
In the digital world, to determine whether 1.000.000 log-lines are generated by 1.000.000 unique devices or rather 100.000 unique devices each generating 10 impressions, you need to be able to tie together the individual log-lines. The de-facto standard for tying together log-lines are cookies, but cookies have massive challenges, which all result in the number of unique visitors being overestimated.
Cookies are not omnipotent identifiers that last forever. Cookies are being deleted all the time – either by users or due to expiration. And when a cookie is deleted, the ability to tie past impressions to future impressions is lost, which result in a new cookie ID being issued to the same device, thus resulting in even more misleading metrics.
One way to illustrate this is by imagining a scenario, where the same device is accessing a website daily for 21 days. In this scenario, there is 1 unique visitor with a frequency of 21. However, if the user’s cookie is deleted after 7 days and again after 14 days, the same device would have 3 different cookie ID’s and the number of unique visitors registered would, therefore, be 3 with a frequency of 7. In other words, both unique visitors and frequency are estimated incorrectly by a factor of 3.
But the challenges with cookies are not only related to the fact that cookies are being deleted. With an increasing share of online users deploying ad-blockers that wipe cookies or straight out refuse to accept cookies at all, a certain percentage of users will lose their cookies as soon as their browsing session ends.
Furthermore, many ad-servers and online analytics services often try to measure frequency and users utilizing their own ID-universe. However, when a third-party cookie system is used for setting and reading cookie ID’s, special precautions need to be taken as iOS devices like iPhones and iPads as well as newer versions of Safari and several versions of Firefox don’t allow third parties to set cookies by default. Thus, a large part of the mobile device population and laptops might not contribute to the visitor estimates.
Moving towards an accurate digital frequency
Our belief is that the data, technology and expert people already exist in order to mathematically and empirically minimise the drawbacks of cookie loss on frequency calculation.
A detailed understanding of online user behaviour and interactions across devices (that’s right, frequency!) can be revealed through a mix of deterministic and probabilistic data inputs, advanced analysis of billions of loglines of data, scale panel data – and importantly, rigorously tested device graphs.
There is no shortage of online data, or indeed algorithms and data scientists – the key though is to get the mix and the method right. Analysts must constantly update the behavioural and panel data at the highest level of quality and regularity, constantly test one’s calculations against a large control data set, to ensure its accuracy and also deliver transparency -black-box frequency estimates aren’t going to wash anymore!
Transparency of method is crucial in order to reassure marketers and publishers that people-based truth-at-scale, is indeed what they are getting, and that frequency is indeed being accurately assessed – because the marketers are certainly going to be looking at the impact on their campaign KPIs that more accurate frequency should deliver.
So, by smartly using what is already available, one can understand if a cookie has been deleted, or if it’s a real, new user – going a long way towards cracking the digital frequency code.
AudienceProject provides full transparency in the data ecosystem and has now launched in the UK. Visit www.audiencedata.com for more.