What is the future of Marketing Mix Modelling?
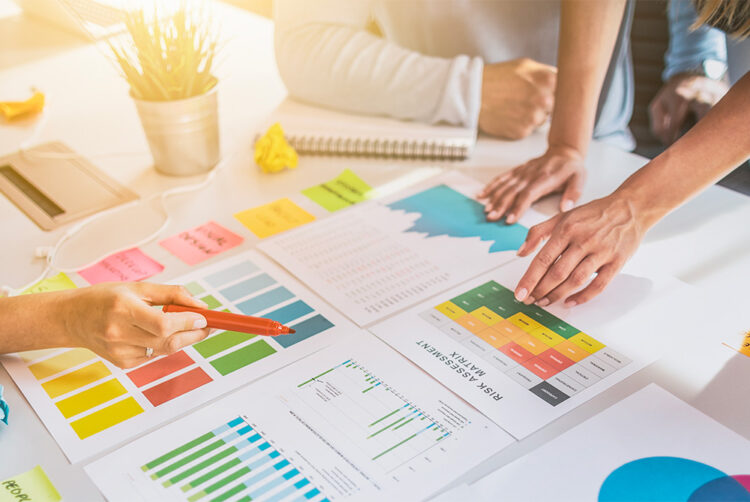
Opinion: Strategy Leaders
Havas’ global managing director of measurement explains why, despite falling out of favour, Marketing Mix Modelling still has a lot to offer modern marketers.
The marketing and advertising industry is a dynamic, ever-evolving organism, and for decades Marketing Mix Modelling (MMM) has been both enabling this transformation and evolving alongside the industry.
MMM analyzes historical data on sales, marketing spending, and other relevant factors such as economic indicators, weather patterns, and seasonality to inform marketing decisions and maximize ROI.
This statistical tool was born in the 1960s when traditional methods of measuring effectiveness such as surveys and focus groups couldn’t keep up with the burgeoning boom of mass media.
As computers became commercially viable in the 1970s, MMM became more accurate and sophisticated, enabling marketing campaigns to scale to new global heights.
In the 1990s and 2000s MMM had to adapt once integrating nascent data sources as digital media began to dominate the ecosystem.
Despite MMM’s decades-long history of delivering marketing accountability, the relevance and effectiveness of this trusted tool have been called into question amidst the rise of digital advertising and the increasing availability of user-level data.
As it has become viable to extract and process vast amounts of data and track user interactions at scale, MMM’s aggregated level of analysis has been labelled as outdated.
However, as history has shown, these new developments do not spell the death of MMM, but rather fuel its continued evolution, and present an opportunity to make this tool more relevant than ever.
Attribution models: cost-effective, but flawed
The waning confidence in MMM can be directly tracked against the rise of another tool in the marketer’s arsenal: third-party cookies.
Third-party cookies enable easy tracking of users across platforms, fuelling low-cost attribution models that can help marketers understand the impact of each touchpoint on the customer journey and assess which actions are driving sales. While these attribution models have gained widespread adoption across the industry, they fall short in many critical areas.
Unlike MMM, attribution models cannot measure incrementality — or the additional revenue generated by a campaign, taking into account the revenue that would have been generated without the campaign — making it difficult to use these models to determine true ROI.
Furthermore, attribution models allocate value to different marketing channels and touchpoints based on a predetermined formula, which raises concerns over the validity of these formulas and the insights that the models generate. When more sophisticated methods are introduced to address this problem, the models often lose the ability to determine which specific touchpoints are generating ROI.
However, perhaps most pressingly, increased privacy concerns and regulations have led to the discontinuation of third-party cookies, which have been the core currency for these attribution models for almost a decade.
In contrast, MMM does not rely on cookies and user-level data, and, with the aid of nascent technology, can provide more accurate insights than attribution models without the associated privacy concerns.
Turbocharging MMM with AI and ML
Historically, one of the pain points of MMM has been the time-consuming and labour-intensive process of inputting data. With the aid of Artificial Intelligence (AI) and Machine Learning (ML), vast amounts of data can be cleaned, organized, and structured in an efficient and accurate manner, allowing the process of data preparation to be automated.
Moreover, MMM requires developing complex statistical models, and selecting the most appropriate algorithm for a given task can be challenging. AI and ML can assist in this area by automating the process of algorithm selection and tuning, ensuring that the most accurate and practical models are used.
On the output side, AI and ML can be used to test and validate the accuracy of MMM models to ensure that results are reliable and accurate. Once the MMM model has been developed and tested, AI and ML can interpret and translate the model into actionable insights, identifying patterns and trends in data, highlighting areas where marketing budgets should be allocated, and providing real-time recommendations for marketers to optimize campaigns.
AI and ML offer unique opportunities when implemented into MMM, as they grow more accurate and faster with increased utilization, allowing models to be scaled to evaluate increasingly complex marketing campaigns and channels. This approach requires a robust infrastructure that includes real-time data streaming, data warehousing, and platforms to support processing and analysis — but the potential for big spenders to adjust marketing campaigns on a monthly, weekly, daily, or even real-time basis offers unparalleled opportunity for optimization.
The future for MMM is bright
The present outlook for MMM is remarkably similar to the 1970s in many ways. Just as computers brought new relevance and scale to MMM in the 70s, AI and ML are poised to revitalize MMM for today’s media landscape, providing the answer for marketers seeking an effective and efficient tool that respects consumer privacy.
The question that marketers and brands should be asking is not whether to implement MMM, but how they can select the best MMM provider.
The best MMM provider is one with an appreciation for MMM’s storied past, but with an eye focused on the future, utilizing new data sources such as location data and the internet of things in conjunction with AI and ML to gain deeper insights into customer behaviour and preferences that be used for real-time decision-making that is accurate, efficient and scalable.
George Papandreopoulos is global managing director of measurement at Havas Media Group.