Your AI initiative is only as good as your expert researcher
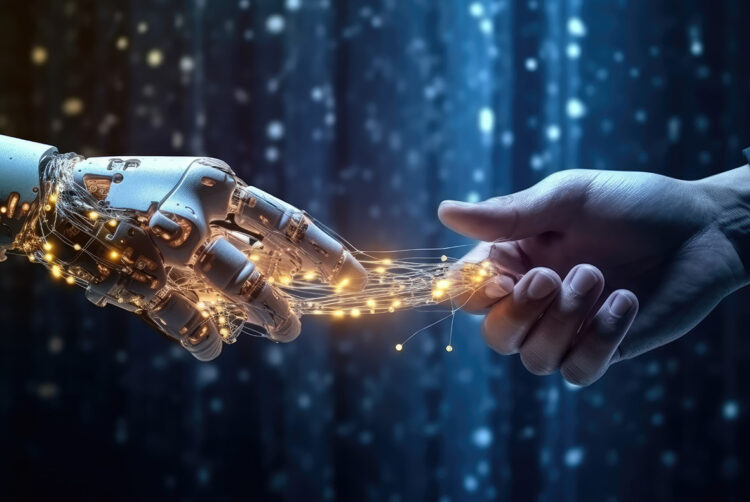
Opinion
For businesses keen to explore AI beyond the obvious use case of generating efficiencies, they require an application of the technology in partnership with human expertise.
Despite the wave of AI evangelism, we don’t yet know how this new technology will reshape the industry.
After all, social media came along 15 years after the world wide web, so it’s equally likely that this new wave of technology will bring unexpected ramifications further down the line.
With a new AI bill expected from Sir Keir Starmer, we can potentially look forward to increased clarity on guardrails in terms of commercial opportunities.
At the same time, pressure to figure out the business opportunity is mounting. According to a survey by Slack, executive urgency to incorporate AI tools into business operations has increased seven times over the past six months and is now a top concern — above inflation and the broader economy.
Experimentation phase
The reality is we are still very much in a phase of experimentation, with perhaps some parts of the industry further ahead than others — for example, creative case studies are much easier to find than media examples.
Brands such as O2, Dove and Heinz have already used AI in their advertising. O2 worked in partnership with VCCP to evolve its brand assets, Dove sought to reassert its message about real beauty and Heinz emphasised the ubiquity of its brand.
In these early stages, very few people can claim to be experts — we’re all testing and learning. In this phase, research practitioners have an important role to play and I’d argue more businesses should be using their natural talents.
This is especially true for businesses that are keen to explore AI beyond the obvious use case of generating efficiencies. If you want to deliver innovation or better outcomes, it requires an application of the technology in partnership with complementary human expertise.
Deep data
AI is powered by data — it needs up-to-date and relevant data to be useful. Furthermore, it can be trained on bespoke data tailored to your objectives when you want to differentiate the outputs from those that are achievable via publicly available sources.
This is exactly what Zappi, a tech-forward market research business, has been doing. It believes in the value of deep data. Huge datasets like social media metrics or clickstream data only get you so far; when you combine these with deep consumer data, you unlock the “why” — which is much more powerful.
Steve Phillips, CEO of Zappi, says: “The key to unlocking AI integration hinges on the data that is used to train it and the synergy between AI and human intelligence. When leveraged correctly, data can be a powerful layer of context that roots AI in the needs of consumers.
“This enables people to spend more time connecting the dots of consumer behaviour and mapping those insights back to the needs of the business.”
The role of researchers
Researchers understand data and, crucially, how to protect its integrity. Clients are already interested in pooling their own data with OpenAI, but any efforts to do so will require careful processes and systems to protect the privacy of their data as well as to assess the usefulness of the outputs.
Specialist software already provides some of the solution, but this type of work also requires good experimental design. Researchers can borrow frameworks such as test-and-control, geo-tests and test-and-learn to help businesses run successful experiments.
We need good-quality experiments so we can understand where and how to use AI — identifying the places where it will add the most value and how it should be used in combination with human intervention.
Challenges ahead
One of the biggest challenges already emerging with AI data is bias. Working with openly available data means you are inevitably replicating all of the existing biases in society when it comes to gender, ethnicity, sexuality and all types of identity and geopolitics.
An awareness of these biases and an understanding of how to restructure data through careful design is essential — this is a core skill of research practitioners.
As well as bias, we need to consider hallucinations. This is where AI runs wild and begins to make things up. This creative inventiveness can be an asset when it comes to idea generation, but it’s less useful when you want to generate ideas grounded in consumer truths.
Being able to spot hallucinations and query outputs and sources is a necessary skill — and one that researchers who are accomplished at representing the voice of the consumer excel at.
Foundation-level skills
Meanwhile, prompt engineering is emerging as a core part of AI infrastructure — a way for us to query and apply, directing its intelligence to particular tasks.
At the heart of generating good prompts is the ability to ask questions, listen and understand, and gradually build up a better picture by asking insightful follow-up questions. As any good qualitative researcher will tell you, this is a foundation-level skill.
There is also a new, emerging discipline around AI ethics — these individuals are positioning themselves as experts at addressing the issue of whether the AI systems we create are aligned with our morals and the values we hold dear as a society.
Researchers — particularly those who focus on sensitive subject matters — have always concerned themselves with such questions, seeing it as their duty not only to advance knowledge but also to protect the human participants.
Working together
It’s inevitable that AI will replace some tasks and jobs in our industry, but I don’t think the researcher has much to fear. Whatever the future holds, it will be some combination of AI and humans working together.
To me, it’s already clear that many of the shortcomings of AI naturally lend themselves to the strengths of research practitioners. I can only see this becoming a partnership that will flourish.
Anna Sampson is a consultant