Finding the signals in the noise of big data
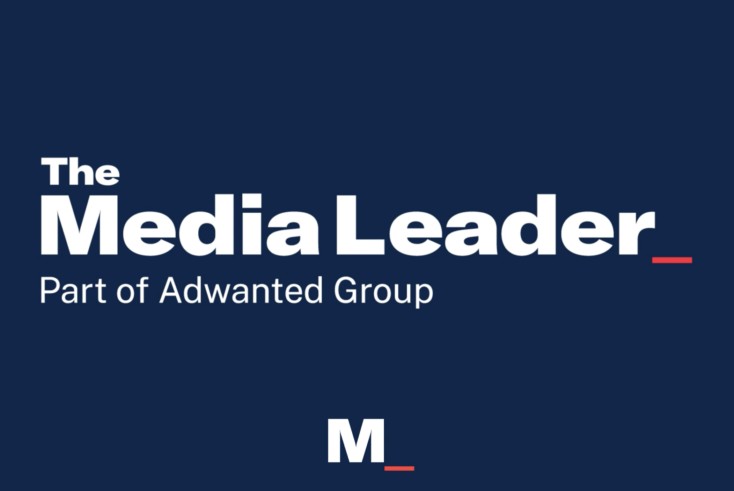
If we are not careful we risk falling victim to the illusion that something is scientific when it has no actual basis in fact, argues GfK’s Colin Strong.
The era of big data has firmly reached the media industry with Sky’s AdSmart and YouView being prime examples of the way in which the traditional broadcast advertising model is being disrupted by data driven technology platforms. Big data surely offers brands real opportunities to enhance their business and drive up both revenues and margin.
But in the midst of the optimism and excitement I often hear media brands expressing concern that they don’t make enough use of the data that is available to them. On the one hand, all too often they stick to the familiar, using traditional metrics which have traction in the business, selecting data points ‘because we can’ rather than because they are sure they are the right ones.
Conversely, brands also seem to assume that simply having swathes of data to analyse will necessarily lead to findings that are grounded in reality; that really make a difference. But simply running off vast numbers of correlations or looking at patterns in the data have their own problems in terms of false positives – an issue that Nate Silver made very clearly some time ago.
For me, the concern here is that if we are not careful we can fumble the big data opportunity and become members of the ‘cargo cult’. By that, I mean falling victim to the illusion that something is scientific when it has no actual basis in fact.
The term comes from a supposedly true story of a group of islanders in the South Seas who watched the American military busily build and maintain airstrips on their islands as bases from which to defend against Japanese attacks following Pearl Harbour.
Without properly considering the context, or really figuring out what questions we want answered, the data we collect can often prove meaningless.”
After the war and the departure of the Americans, the islanders wanted to continue to enjoy all the material benefits the American airplanes had brought: the ‘cargo from the skies’. So they built replica runways, a wooden hut and wooden headset for their version of a controller in the hope that it would all return.
But, of course, the airplanes never came, even though the islanders went about it ‘scientifically’. In other words, the data they used an as input was flawed.
The moral of this story is that without properly considering the context, or really figuring out what questions we want answered, the data we collect can often prove meaningless. Of course, as Alistair Croll and Benjamin Yoskovitz point out in their book Lean Analytics, it’s far too easy to fall in love with ‘vanity’ data points.
These are the ones that consistently move up and make us feel good but really don’t help us make decisions that affect actual performance. Well known examples of these could include number of hits, visits, followers, friends, likes, time spent on site, etc. All cases where the data collected often (but not always) bears no real relationship to the success or otherwise of the business model.
So we need to find ways to help us sift out these vanity metrics, helping us to organise our thinking to navigate the mass of data and avoid joining the ‘Cargo Cult’. Fortunately, there are a number of frameworks available to help with this process of identifying what the appropriate data should be for your own organisation to help meet your particular goals. One such framework comes from the former US Secretary of Defence Donald Rumsfeld who famously said:
“… there are known knowns; there are things we know that we know. There are known unknowns; that is to say, there are things that we now know we don’t know. But there are also unknown unknowns – there are things we do not know we don’t know.”
He made his comment at a press briefing in 2002, where he addressed the absence of evidence linking the government of Iraq with the supply of weapons of mass destruction to terrorist groups. His somewhat unusual phrasing got huge coverage, to the extent that he used it as the title of his subsequent autobiography, Known and Unknown: A Memoir (Rumsfeld, 2012).
Media brands are often left struggling to determine the best way to navigate the immense oceans of data at their disposal.”
Opinions were divided about his comments. For instance, it earned him the 2003 Foot in Mouth Award and was criticised as an abuse of language by, among others, the Plain English Campaign.
However, he had his defenders – among them Croll and Yoskovitz, who made good use of Rumsfeld’s phrase to design a way of thinking about data. Their view is that analytics have a role to play in all four of Rumsfeld’s quadrants:
– Things we know we know (facts). Data which checks our assumptions – such as open rates or conversion rates. It’s easy to believe in conventional wisdom that ‘we always close 50% of sales’, for example. Having hard data tests the things we think ‘we know we know’.
– Things we know we don’t know (questions). Where we know we need information to fill gaps in our understanding.
– Things we don’t know we know (intuition). Here the use of data can test our intuitions, turning hypotheses into evidence.
– Things we don’t know we don’t know (exploration). Data which can help us find the nugget of opportunity on which to build a business.
There is something quite appealing about this approach, not least because of the way it engages an audience to think about the different challenges of data. It also introduces the concept of exploratory analysis, an important distinction to make but one which is often confused.
‘Reporting’ data points support the business to optimise its operation to meet the strategy while ‘exploratory’ data points set out to find the nugget of opportunity. These “unknown unknowns” are where the magic lives. They might lead down plenty of wrong paths, but hopefully toward some kind of “Eureka!” moment of a brilliant idea that disrupts markets.
Nevertheless, in any kind of business, both types of data analytics are of course essential. In smaller start-ups the balance will often be titled more towards the ‘things we don’t know’ while in more established businesses there may be more focus on measuring the ‘things we know’. But any business ignores either side of this at its peril.
Media brands are often left struggling to determine the best way to navigate the immense oceans of data at their disposal. By taking a more structured approach to the design of the data analytics process, brands can avoid becoming members of the Cargo Cult, with all the business implications that can lead to.
Colin Strong is managing director at GfK NOP Business & Technology.
To get all the latest MediaTel Newsline updates follow us on Twitter.